COVID-19 and Haters — A User Model Perspective
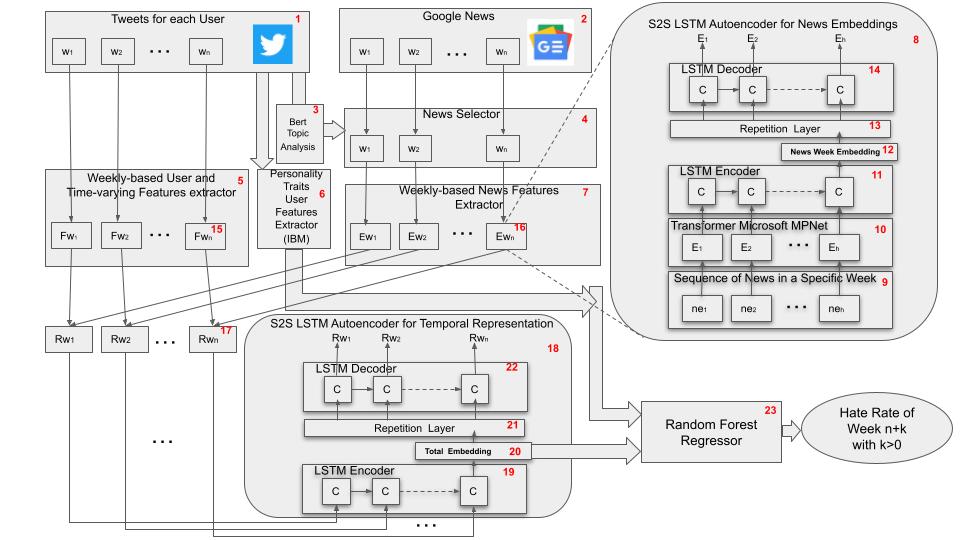
We present an in-depth analysis of users' propensity toward negative and hateful behavior during the COVID-19 pandemic. We analyze a large dataset extracted from Twitter from the months of January 2020 up until June 2020. The dataset includes 2,470,888 tweets from 3,269 users who are active over a period of six months. We model users' propensity toward hateful content over time by leveraging Random Forest regressor model and Long Short-Term Memory (LSTM) based many-to-one and Sequence2Sequence models for both short and long-term predictions. Our models leverage a set of features for each user, including the user's psychological traits. We also study the impact of external triggers, such as COVID-related news concurrent with the users' activities. To encode popular news, we propose using encoder states of a Sequence2Sequence model as features for a Tree-based regressor. The regressor, when combined with the vectorized news, results in an accurate prediction of tweeter's hateful behavior in the short (decoder size of four weeks) and long term (decoder size of 10 weeks) with a total training data of 15 weeks x 3269 users. We also show that our model accurately profiles selected groups of users, as they are defined by specific psychological traits.
Publications
- Soumitra Mehrotra, Edoardo Serra, Anna Squicciarini, Younes Karimi;
COVID-19 and Haters—A User Model Perspective, IEEE DSAA, 2022
Paper | Slides | Codes